How AI Is Reshaping Language Revitalization
By Sofia Osborne
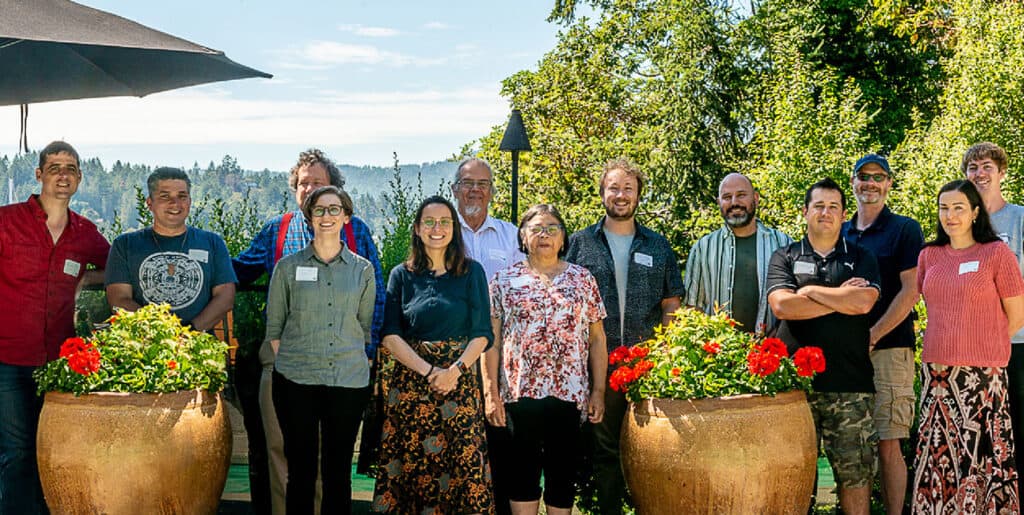
When Aidan Pine was in the first year of his linguistics degree at the University of British Columbia, he wanted to get experience working with language revitalization outside his coursework. He joined a research project, working with speakers to create a dictionary for the Gitksan language, spoken by the Gitksan Nation of British Columbia, which the researchers hoped ultimately to make into an app. But, Pine says, they quickly realized how challenging it was to get that kind of technology made by a non-Indigenous company.
“There were a lot of companies, [but] they didn’t really understand the issues that surrounded these types of dictionaries,” he says. “They had expertise in building websites generally, but they didn’t know how to work with Indigenous languages.”
The companies were also quoting large exorbitant amounts of money, up to nearly $100,000, to produce the app.
“We put years of work into this… why isn’t there an easier way to publish it?” Pine recalls asking himself.
When it comes to adopting technology for language learning, there are other important factors to consider, like data privacy and whether communities retain ownership of the material they upload. So, taking matters into his own hands, Pine made the Gitksan app as his undergraduate thesis project.
He soon realized this type of software could also be useful for other Indigenous communities seeking to mobilize the language data they were collecting, and so his organization, Mother Tongues, was born.
Free and open source, Mother Tongues Dictionaries allows communities to create their own, easy-to-use dictionary apps for their languages, available on iOS, Android, and the web. Crucially, once downloaded, the apps can be used offline.
Pine says one of the most important features he wanted to include in the Mother Tongues software is an approximate search algorithm, which returns words that are similar to but not exactly the same as those the user has searched for.
“The majority of people who use these dictionary apps are learners, and so there was this frustrating experience when the search algorithm that they were using was very rigid—you had to spell the words exactly as they were written in the dictionary,” Pine says. “So I was a learner and in order to look up a word, I had to already know how to spell it. Which is a bit of a paradox, right?”
Currently, there are more than 20 linguistic communities using Mother Tongues for their dictionaries. Pine says he recommends educators take a look on the App Store or on their website to see if a dictionary of the language they are teaching is available for download.
Pine is also a research officer with the National Research Council of Canada, where he works on the Indigenous Languages Technology Project, an initiative whose mandate is to create software to help Indigenous language revitalization.
“Our philosophy was not to try to push technologies that we thought might be useful or interesting onto Indigenous communities but rather to ask them what they would find useful,” says Roland Kuhn, the head of the project.
One of the team’s most popular sub-projects is the ReadAlong Studio, a tool that allows educators to create interactive, read-along stories that feature automatically synchronized text and speech.
“What we found when we were talking with a lot of educators, curriculum developers, and students, was that there was a real bottleneck for creating audio and text educational content in Indigenous languages,” Pine says. “A lot of the teachers maybe had some text and they maybe had some corresponding audio… but combining those two things in an educationally accessible format was a real challenge.”
The ReadAlong Studio software uses a form of speech recognition called text audio alignment, which automatically matches up speech with the corresponding word in the story. Read-alongs are created by pairing a text with an Elder reciting the story in an Indigenous language. Those trying to learn that language are then able to follow along, slow down, and play back certain words to hear their proper pronunciation.
Courtesy of Dr. M. Jane Smith (Xsiwis) and Michelle Stoney, Mother Tongues, and the Gitksan Research Lab Courtesy of Mother Tongues, the Gitksan Research Lab, and Ken Mowatt (Maaslik’i’nsxw)
While the tool was developed to be used for Indigenous languages, it is highly flexible. So far, read-alongs have been created by educators from countries around the world, among them Colombia, Nepal, the Netherlands, Nigeria, and Taiwan.
Another tool created by the Indigenous Languages Technology Project is a verb conjugator for Kanyen’kéha, also known as Mohawk, which was suggested to the team by Kanyen’kéha educator Owennatekha.
When the team asked Owennatekha what would be most helpful for the students at the Onkwawenna Kentyohkwa school (Our Language Society) he founded at Six Nations of the Grand River, he said learners struggle most with verbs. Kanyen’kéha is a polysynthetic language, in which single words that are combinations of smaller elements of meaning, called morphemes, can often be equivalent to full sentences in languages like English. Kanyen’kéha is dominated by verbs, which are very often used to express things that might be expressed as nouns in other languages.
At the Onkwawenna Kentyohkwa school, instructors use a root-word method to help students learn and combine these morpheme building blocks.
Kuhn and his team worked with Owennatekha and other teachers at the school to create the verb conjugator software, an interactive program that allows users to select the subject (person), tense (time), and verb (action), and press a button to receive the correct conjugated form of the verb. While classroom time is focused on interaction between instructors and students, Owennatekha says the verb conjugator serves as a helpful reference.
“Trying to memorize a language like Kanyen’kéha is the same thing as trying to learn English by memorizing whole sentences,” Owennatekha says. “So that’s the beauty of our [verb conjugator] method.”
While Indigenous languages spoken in Canada are extremely diverse, they are almost all polysynthetic. When other Indigenous language educators in Canada saw the verb conjugator prototype, they started requesting it for their own languages. Since then, members of the team have created conjugators for Michif, Mi’kmaq, and Anishinaabemowin, and are working on interactive apps for Nêhiyawêwin and SENĆOŦEN.
“That prototype is an example of a project that was originally suggested by an educator who teaches Kanyen’kéha, but which turned out to be very useful for other Indigenous languages spoken in Canada, even [linguistically] unrelated ones,” Kuhn says. “That’s what we’re always looking for.”
Now, Pine, Kuhn, and others from the Indigenous Languages Technology Project are working with Onkwawenna Kentyohkwa to bring the words produced by the verb conjugator to life.
“It’s one thing to be able to get this app to create a verb, but you look at it and it’s just gobbledygook to people who don’t know the language,” Owennatekha says.
The team is working on using text-to-speech technology to create a tool that will allow learners to hear the pronunciation of words they produce. With hundreds of thousands of possible conjugations, it would take years and a tremendous amount of work to have speakers record each pronunciation manually. Instead, a neural network—a set of interconnected artificial nodes made to model the human brain—can be trained on the relationship between text and speech using data from recordings of audiobooks, speeches, stories, and other sources. Through trial and error, Pine realized it only takes a few hours of data for the algorithm to be able to provide pronunciations that are accurate enough for teaching.
Owennatekha also hopes the text-to-speech software can be implemented for the textbooks used at the school.
“[Students] would ideally be able to click on a word and hear it pronounced,” he says. “That would really be an enormous help.”
The text-to-speech model is one of very few projects involving Indigenous languages spoken in Canada that uses artificial intelligence in the contemporary sense of the word, Kuhn explains. This is because there is a lack of data available, making them what linguists call low resource languages.
“People who only speak English or French don’t realize that for those [major European] languages, there’s tons of free data floating around on the web,” Kuhn says. “That’s not the case for Indigenous languages spoken in Canada, or indeed for most languages.”
To date, Inuktitut is the only Indigenous language spoken in Canada for which successful machine translation projects have been produced, as there is far more parallel data available for the Inuktitut-English language pair than for other languages. This is in great part thanks to the proceedings of the Nunavut Legislative Assembly, which are written in Inuktitut in parallel with English.
Kuhn and his team worked with the Nunavut Legislative Assembly to match up 1.3 million Inuktitut sentences with their English translations. They released this parallel corpus in 2020, and the team and other researchers use it to train machine translation systems.
Still, Kuhn says the quality of the systems is not great on average.
This “1.3 million sentence pairs isn’t enough to train a high-quality machine translation system, especially when the two languages involved, English and Inuktitut, have such different grammatical structures,” he says. “A system trained on 13 million sentence pairs, or 130 million, would be much better.”
By contrast, Kuhn says it would be hard to find even 20,000 language pairs between Kanyen’kéha and English.
“With so little training data, it’s impossible to build a decent system for translating between these two languages,” he says. “Fortunately, our Mohawk collaborators aren’t interested in machine translation from English, as most, if not all Mohawks are fluent in English. Instead [that] collaboration focuses on software to help learners of the language on creating educational software to help learners speak and write in their ancestral language.”
This lack of data also poses a challenge for low resource languages around the world, something that researchers like Ife Adebara—a PhD candidate in the Department of Linguistics at the University of British Columbia who works on AI tools for African languages—are trying to address.
“I know a lot of English speakers take it for granted that we have access to the web, and we can do whatever we want to do in English and have a lot of tools and resources,” she says. “But for people who do not speak English, and who speak a lot of these less widely spoken languages, they are completely cut out of that whole technological advancement.”
Adebara created a language identification tool called AfroLID, which uses AI to identify 517 African languages and dialects from any given text. A model like this is important, Adebara explains, as it allows researchers to find data around the web that can be used to train AI. In this way, AfroLID is a stepping stone towards developing Natural Language Processing (NLP) systems for these African languages that will be able to process language in the same way humans do.
Predictably, one of the biggest hurdles Adebara faced in creating AfroLID was a lack of data. There are few documents available in, and few researchers working on, these languages.
“If you are working on NLP in English, you can crawl any of the news stations and you will get a lot of texts daily on the web in English,” she says. “It takes a lot more effort and intentionality to find anything from these [African] languages.”
Ultimately, it took Adebara’s team over two years to curate the data set they used to create AfroLID. After this experience, she is trying to encourage policy changes that will ensure data is available in African languages.
“In Nigeria, where I come from, education is in English, so that already cuts out the level of literacy in these other languages,” she says. “This filters down to the availability of data. And so there are a lot of policy issues that need to be changed.”
With AfroLID complete and able to collect data and detect which language it is in, Adebara and her research group have moved on to other AI projects. This summer, they launched Serengeti, a language understanding model that learns general information about a language and can be used to complete tasks like identifying key names and nouns in a document. A tool like this means that speakers of these 517 African languages could use a search engine in their own language.
Adebara says that there can be a lot of pressure to learn a widely spoken language in order to have access to more resources, but that can hinder the use of the languages a person already knows. “People might begin to feel that their languages are not important, and that some other major languages are more important than theirs. And that has a psychological effect.”
If more data can be collected and identified using AfroLID, and more AI models can be generated using that data, Adebara hopes machine translation between English and these African languages will be possible, opening the door for more people to access resources like textbooks.
Ultimately, Adebara says, this work is important in diversifying the AI space.
“When people don’t have access [to technology] in the languages that they speak, we actually lock them out from global conversations,” she says. “We don’t hear their perspectives on the issues that we’re dealing with. And they don’t hear our perspectives either… If we have access to more people’s opinions and perspectives about global issues, perhaps we’ll be able to solve some of these issues a lot faster than we are right now.”
Photos courtesy of Onkwawenna Kentyohkwa
Right: Owennatekha (standing, left) is Program Co-ordinator and Instructor at Onkwawenna Kentyohkwa, a community-based organization that teaches Kanyen’keha for adults
From tools being created to help revitalize Indigenous languages spoken in Canada to AI models that bring access to people who speak the world’s 7,000+ languages, technology is changing the way we work with language. Still, Kuhn says, it is speakers and learners who are at the heart of it all.
“[The educators] are the directors, the playwrights,” he says. “We’re like stagehands. We help with the lighting. Maybe we can make the scene a little bit better… But we’re not the centre of the story. The centre of the story is the educators who are trying to bring their ancestral languages back.”